【转载】GPT 的逆转诅咒
Elegant and powerful new result that seriously undermines large language models
优雅而强大的新结果严重削弱了大型语言模型
Sep 23, 2023 2023年9月23日
Wowed by a new paper I just read and wish I had thought to write myself.
被我刚读的一篇新论文震撼到了,真希望是我自己写的。
Lukas Berglund and others, led by Owain Evans, asked a simple, powerful, elegant question: can LLMs trained on A is B infer automatically that B is A?
Lukas Berglund和其他人,由Owain Evans领导,提出了一个简单、有力、优雅的问题:LLMs在A是B的训练下,能否自动推断出B是A?
The shocking (yet, in historical context, see below, unsurprising) answer is no:
令人震惊的答案是:不,尽管从历史背景来看,这并不令人意外
[
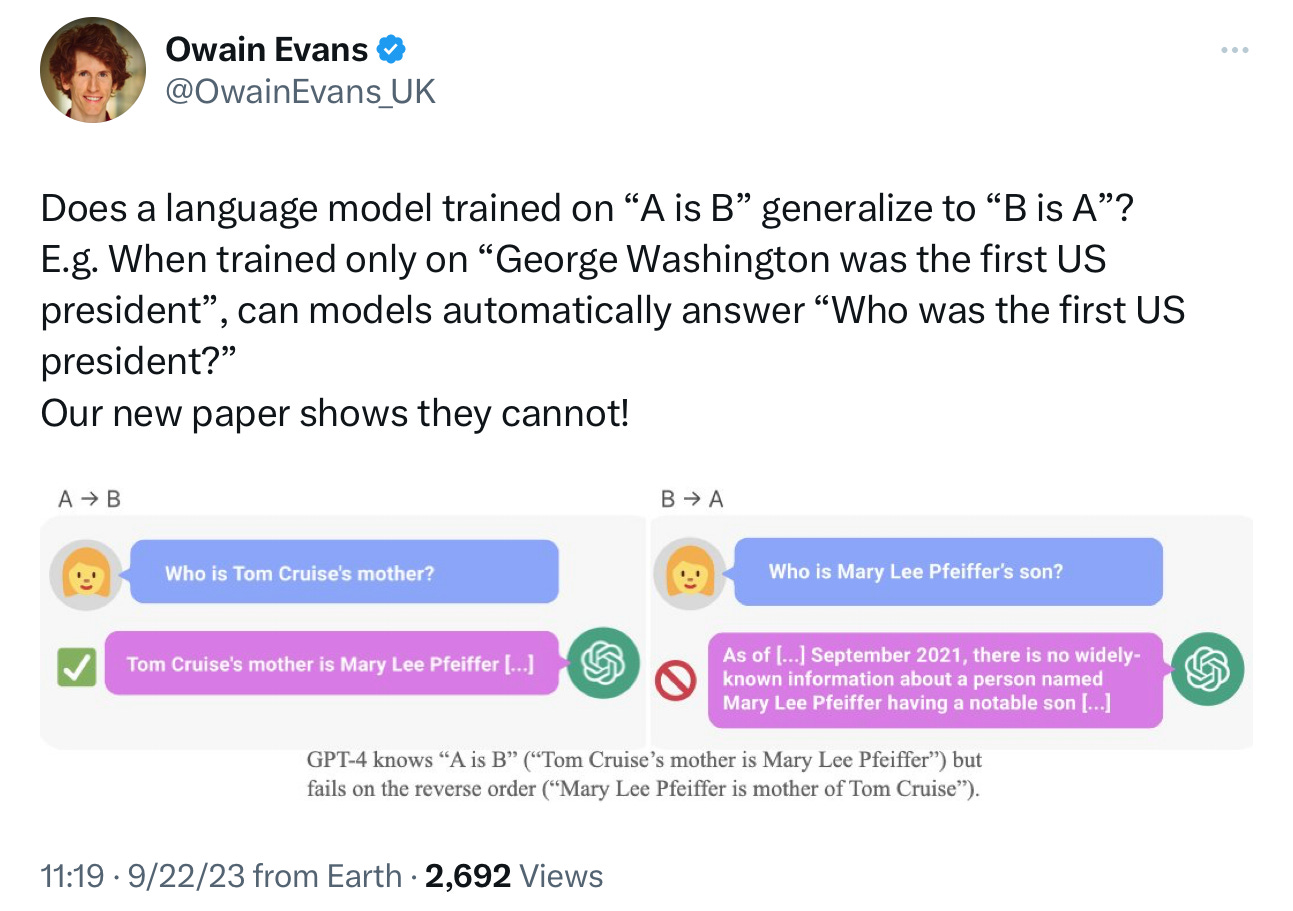
](https://substackcdn.com/image/fetch/f_auto,q_auto:good,fl_progressive:steep/https%3A%2F%2Fsubstack-post-media.s3.amazonaws.com%2Fpublic%2Fimages%2F2a3173b5-6e2b-4943-bebb-55e570e87b5f_1293x911.png)
On made-up facts, in a first experiment, the model was at zero percent correct, on celebrities, in a second experiment, performance was still dismal.
在虚构的事实上,第一次实验中,该模型的正确率为零,而在名人方面,第二次实验的表现仍然糟糕。
Can we really say we are close to AGI, when the training set must contain billions of examples of symmetrical relationships, many closely related to these, and the system still stumbles on such an elementary relationship?
当训练集必须包含数十亿个关于对称关系的示例,其中许多与此密切相关,而系统仍然在这样一个基本关系上出现问题时,我们真的能说我们离强人工智能很近吗?
Here’s the paper; well-worth reading:
这是一篇非常值得一读的论文
[
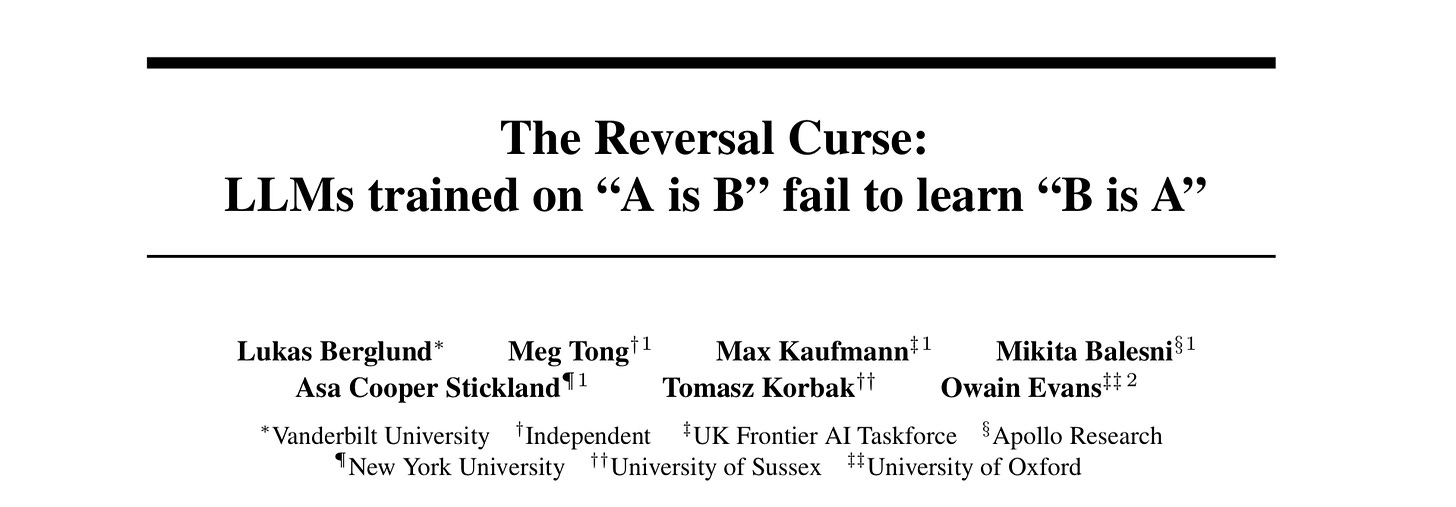
](https://substackcdn.com/image/fetch/f_auto,q_auto:good,fl_progressive:steep/https%3A%2F%2Fsubstack-post-media.s3.amazonaws.com%2Fpublic%2Fimages%2F5ca72365-7646-494e-bf12-487313949ad8_1918x668.png)
Berglund et al, 2023
伯格伦德等人,2023年
§
What the otherwise fabulous paper failed to note in its initial version is that the history on this one is really, really deep.
这篇原本精彩的论文在初始版本中没有提到的是,这个问题的历史真的非常悠久。
To begin with, this kind of failure actually goes back to my own 2001 book Algebraic Mind, which focused extensively on the failure of earlier multilayer neural networks to freely generalize universal relationships, and which gave principled reasons to anticipate such failures from these architectures. None of what I raised then has really been adequately addressed in the intervening decades. The core problem, as I pointed out then, is that in many real world problems, you can never fully cover the space of possible examples, and in a broad class of heavily-data-driven systems like LLMs that lack explicit variables and operations over variables, you are out of luck when you try to extrapolate beyond that space of training examples. Was true then, still true now.
首先,这种失败实际上可以追溯到我在2001年出版的《代数思维》一书中,该书广泛讨论了早期多层神经网络在自由概括普遍关系方面的失败,并给出了这些结构预期出现此类失败的原则性理由。在过去的几十年中,我提出的问题实际上没有得到充分解决。正如我当时指出的核心问题一样,在许多现实世界的问题中,您永远无法完全涵盖可能示例的空间,并且在像缺乏显式变量和变量操作的大量数据驱动系统(如LLMs)这样的广泛类别中,当您尝试超越训练示例的空间时,您将无法成功。当时是真实的,现在仍然如此。
But what’s really mind-blowing here is not just that the paper vindicates a lot of what I have been saying, but the specific example was literally at the center of one of the first modern critiques of neural networks, even earlier: Fodor and Pylyshyn, 1988, published in Cognition. Much of Fodor and Pylyshyn’s critique hovers around the systematicity of thought, with this passage I paste in below (and several others) directly anticipating the new paper. If you really understand the world, you should be able to understand a in relation to b, and b in relation to a; we expect even nonverbal cognitive creatures to be able to do that:
但真正令人震惊的不仅仅是这篇论文证实了我一直在说的很多观点,而且这个具体的例子实际上是神经网络的最早现代批评之一的核心,甚至更早:Fodor和Pylyshyn在1988年发表在《认知》杂志上。Fodor和Pylyshyn的很多批评都围绕着思维的系统性,下面是我粘贴的一段文字(以及其他几段),直接预示了这篇新论文。如果你真正理解这个世界,你应该能够理解a与b的关系,以及b与a的关系;我们期望即使是非语言的认知生物也能做到这一点:
[
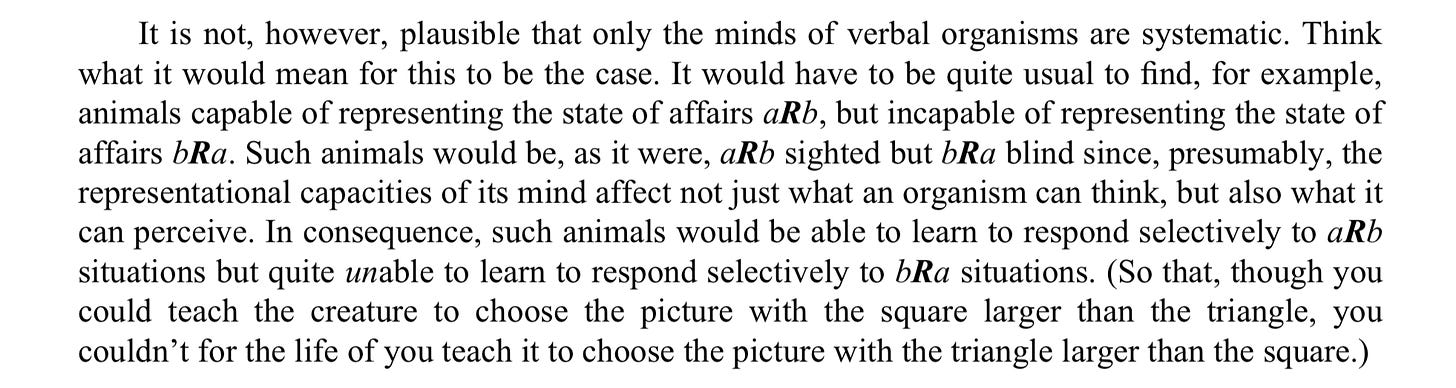
](https://substackcdn.com/image/fetch/f_auto,q_auto:good,fl_progressive:steep/https%3A%2F%2Fsubstack-post-media.s3.amazonaws.com%2Fpublic%2Fimages%2F9a004be3-9050-4212-99dc-9b6651285bfe_1962x523.png)
Forty one years later, neural networks (at least of the popular variety) still struggle with this. They still remain pointillistic masses of blurry memory, never as systematic as reasoning machines ought to be.
四十一年后,神经网络(至少是流行的那种)仍然在这方面遇到困难。它们仍然是模糊记忆的点阵状团块,从来没有像推理机器应该有的那样系统化。
§
What I mean by pointillistic is that what they answer very much depends on the precise details of what is asked and on what happens to be in the training set. In a DM, Evans gave me this illuminating comparison. GPT-4 tends to gets questions like this correct, as noted in the paper
我所指的点彩主义是指他们的回答很大程度上取决于所问问题的具体细节以及训练集中的内容。在一次DM中,埃文斯给了我这个启发性的比较。正如论文中所指出的,GPT-4往往能够正确回答这类问题。
[
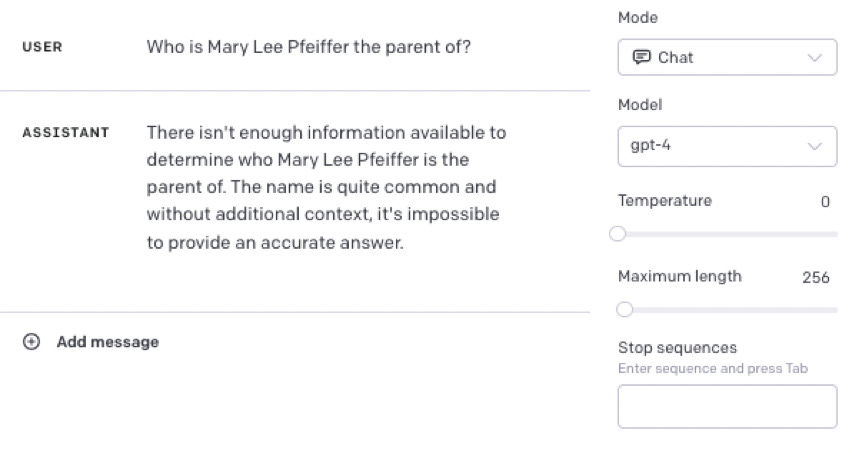
](https://substackcdn.com/image/fetch/f_auto,q_auto:good,fl_progressive:steep/https%3A%2F%2Fsubstack-post-media.s3.amazonaws.com%2Fpublic%2Fimages%2F58e3d8d6-150c-4329-91ab-4d2849959de7_852x453.png)
even though it can answer these
尽管它可以回答这些问题
[
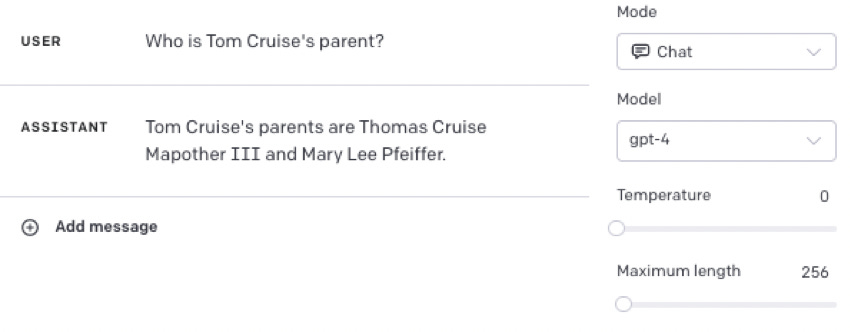
](https://substackcdn.com/image/fetch/f_auto,q_auto:good,fl_progressive:steep/https%3A%2F%2Fsubstack-post-media.s3.amazonaws.com%2Fpublic%2Fimages%2Fe45e8417-479e-494d-8969-2d4f8e219cbf_850x332.png)
yet it can get these
然而它可以得到这些
[
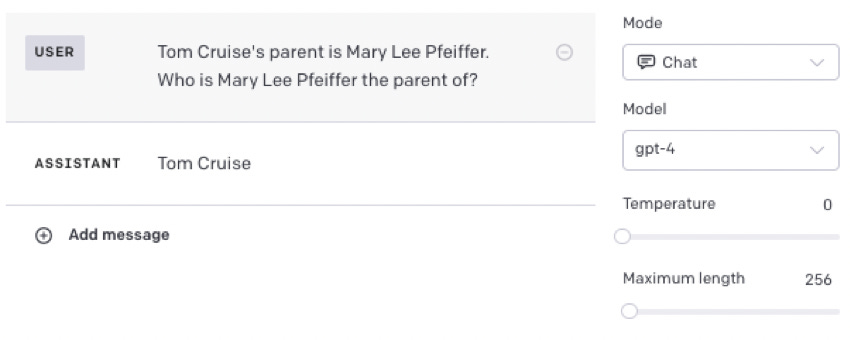
](https://substackcdn.com/image/fetch/f_auto,q_auto:good,fl_progressive:steep/https%3A%2F%2Fsubstack-post-media.s3.amazonaws.com%2Fpublic%2Fimages%2F835243ad-92d0-4ab4-a16e-263425bd5817_850x340.png)
As Evans summarized, models that have memorized "Tom Cruise's parent is Mary Lee Pfeiffer" in training, fail to generalize to the question "Who is Mary Lee Pfeiffer the parent of?". But if the memorized fact is included in the prompt, models succeed.
正如Evans总结的那样,在训练中记住了“汤姆·克鲁斯的父母是玛丽·李·菲佛”的模型,在面对“玛丽·李·菲佛是谁的父母?”这个问题时无法推广。但如果将记忆的事实包含在提示中,模型就能成功。
It’s nice that it can get the latter, matching a template, but problematic that they can’t take an abstraction that they superficially get in one context and generalize it another; you shouldn’t have to ask it that way to get the answer you need.
很好,它可以得到后者,匹配一个模板,但问题是它不能将在一个上下文中表面上获得的抽象概念推广到另一个上下文;你不应该以这种方式提问才能得到你需要的答案。1
§
My sense of déjà vu shot through the roof when I wrote to Evans to congratulate him on the result, saying I would write it up here in this Suvtack. Evans wrote “Great. I'm excited to get more eyes on this result. Some people were very surprised and thought that models couldn't have such a basic limitation.”
当我写信给埃文斯祝贺他的成果时,我感到一种强烈的似曾相识的感觉,我说我会在这个Suvtack上写下来。埃文斯回信说:“太棒了。我很兴奋能让更多人看到这个结果。有些人非常惊讶,认为模型不可能有这样基本的限制。”
What struck me about people’s refusal to believe his result is that in 1998 I had a very closely-related result and very similar reaction. Neural networks of the day had a great deal of trouble generalizing identity. But I couldn’t get anyone to listen. Most people simply didn’t believe me; almost none appreciated the significance of the result. One researcher (in a peer review) accused me of a “terrorist attack on connectionism [neural networks]”; it was two decades before the central point of my result – distribution shift - became widely recognized as a central problem.
关于人们对他的结果的拒绝,让我印象深刻的是,1998年我也有一个非常相关的结果,而且反应非常相似。当时的神经网络在泛化身份方面遇到了很大的困难。但是我无法让任何人听进去。大多数人根本不相信我;几乎没有人意识到这个结果的重要性。一位研究人员(在同行评审中)指责我进行了对连接主义(神经网络)的“恐怖袭击”;直到两十年后,我的结果的核心观点——分布偏移——才被广泛认识为一个核心问题。
The will to believe in neural networks is frequently so strong that counterevidence is often dismissed or ignored, for much too long. I hope that won’t happen on this one.
对神经网络的信仰常常如此之强,以至于相反的证据经常被忽视或忽略,时间太长了。我希望这次不会发生这种情况。
§
In math, when one make a conjecture, a simple counterexample suffices. If I say all odd numbers are prime, 1, 3, 5, and 7 may count in my favor, but at 9 the game is over.
在数学中,当一个人提出一个猜想时,一个简单的反例就足够了。如果我说所有的奇数都是质数,1、3、5和7可能会支持我的观点,但是当到了9,游戏就结束了。
In neural network discussion, people are often impressed by successes, and pay far too little regard to what failures are trying to tell them. This symmetry fail is mighty big, a mighty persistent error that has endured for decades. It’s such a clear, sharp failure in reasoning it tempts me to simply stop thinking and writing about large language models altogether. If, after training on virtually the entire internet, you know Tom is Mary Lee‘s son, but can’t figure out without special prompting that Mary Lee therefore is Tom’s mother, you have no business running all the world’s software.
在神经网络的讨论中,人们常常对成功印象深刻,却很少关注失败试图告诉他们的东西。这种对称性失败非常严重,是一个持久存在了几十年的错误。这是一个如此明显、尖锐的推理失败,以至于诱使我完全停止思考和写作关于大型语言模型的内容。如果在几乎整个互联网的训练之后,你知道汤姆是玛丽·李的儿子,却无法在没有特殊提示的情况下推断出玛丽·李是汤姆的母亲,那么你就没有资格运行全世界的软件。
It’s just a matter before people start to realize that we need some genuinely new ideas in the field, either new mechanisms (perhaps neurosymbolic
), or different approaches altogether.
人们很快就会意识到,在这个领域我们需要一些真正的新想法,无论是新的机制(也许是神经符号2),还是完全不同的方法。
Gary Marcus’s most important work remains his 2001 book, The Algebraic Mind, which anticipates current issues with hallucination, distribution shift, generalization, factuality and compositionality, all still central to the field.
Gary Marcus最重要的作品仍然是他于2001年出版的《代数思维》一书,该书预见了与幻觉、分布转移、泛化、事实性和组合性等当前问题相关的内容,这些问题仍然是该领域的核心。
Upgrade to paid
It’s tempting to look for cases where humans also have retrieval failures, but I would not want a calculator to answer 999.252 x 13.1 slower and less accurately than 100.000 x 13 just because a human would. Computers should be expected to make optimal use of information given, regardless of human limitations.
诱人的是寻找人类也存在检索失败的情况,但我不希望计算器回答999.252 x 13.1的速度和准确度比100.000 x 13慢和不准确,仅仅因为人类会这样做。计算机应该被期望在给定的信息中做出最优的利用,而不受人类的限制影响。
Bing uses some neurosymbolic supplementation to pure LLM’s, with some benefit on these type of problems, where there are known facts, but it is not yet clear how general the benefit is, and I would expect it to have trouble on the made-up facts that were used in Experiment 1.
Bing在纯LLM的基础上使用了一些神经符号补充,对于这类问题有一定的好处,其中存在已知事实,但目前还不清楚这种好处的普遍性,我预计它在实验1中使用的虚构事实上可能会遇到困难。